This is the next in a line of periodic interviews with Fluxnet Young Scientists. This month, we’re glad to introduce Ke Xu, who is a PhD student in the University of Wisconsin, Madison, USA. Ke recently published an interesting paper in Agricultural and Forest Meteorology, where she targeted one of the still challenging issues in eddy covariance measurements, i.e., the footprint representativeness (see followings for details). More information on Ke is available through her ResearchGate Link.
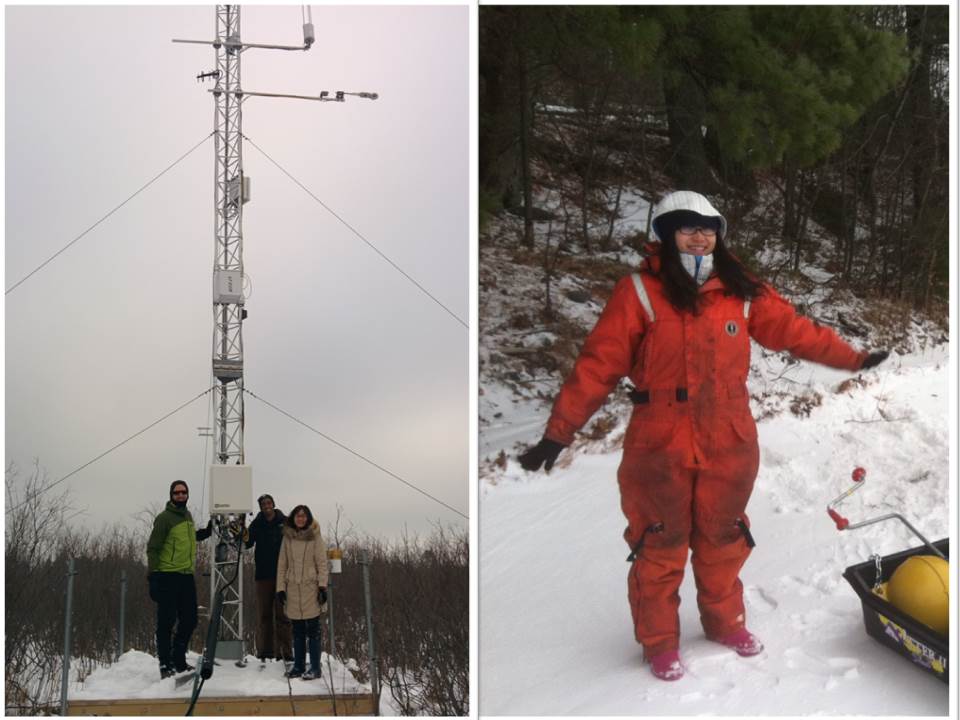
Please tell us about yourself :
My first research experience was working with Prof. Weimin Ju at the Nanjing University, China, where I earned my bachelor degree in geographical information system and remote sensing. My bachelor thesis focused on retrieving the subcanopy reflectivity using multi-directional remote sensing data. Those earlier experiences intrigued me to see how the truth could be unveiled by scientific exploration and investigation and to learn how scientific results can be conveyed in a story-like way. Afterwards, I continued my research journey and started to pursue my PhD degree at the University of Wisconsin-Madison in 2012, where I met Prof. Ankur R Desai. He is an innovative, encouraging and super-efficient supervisor. At first, I was working on the project studying CH4 flux over heterogeneous landscapes in North Wisconsin. However, in summer 2013, my dear supervisor sent me to the Fluxcourse in Boulder, CO, USA, at which a new world of eddy covariance was unlocked in front of me. Through this flux course, I learned the theoretical basics, hands-on installation, and simple data processing of eddy covariance measurements. Later in the summers of 2013 and 2014, I got a great opportunity to intern at the National Ecological Observatory Network (NEON), working with my other mentor, Dr. Stefan Metzger, a staff scientist in NEON and an expert in micrometeorology. Working with Stefan not only furthers my knowledge on processing and interpreting the eddy covariance data, but also helps me to learn organize and prioritize my research in a better and more beautiful way. All these experiences have inspired me and led me to the path of studying land-atmosphere interactions using eddy-covariance measurements, and to where I am now.
Please tell us about your recent studies. What’s the main take-home message?
Despite decades of applications worldwide, eddy covariance technique still confronts a number of challenges especially when attempting to measure surface-atmosphere exchanges in less ideal conditions. Among those challenges, footprint bias and energy imbalance are two major ones. The footprint of eddy covariance towers varies temporally and is usually within the areas of 0.1–10 km2, which is far smaller than the canopy scale and the resolution of most earth system models (100–1000 km2), causing a scale mismatch in model-data comparison. Furthermore, almost all flux towers suffer from imbalanced energy budget, a problem that the sum of measured sensible and latent heat flux is about 20% less than the net radiation minus ground heating.
To mitigate the footprint bias, our recent work (Xu, Metzger, and Desai, 2017) developed an upscaling approach, the environmental response functions (ERFs), to map surface-atmosphere exchange to a 20 km × 20 km region around flux towers. The underlying principle firstly relates measured fluxes and environmental drivers, e.g. meteorological drivers and biophysical surface properties, and utilizes the extracted relationship for spatial and temporal mapping. Consider the metaphor of the Blind Man and the Elephant: the tower (“the blind man”) can describe only one part of “the elephant” of ecosystem fluxes at a time, namely those fluxes that arise from the flux footprint influence area. If the “elephant” were stationary, then over time, the time-varying footprint would allow the tower to map fluxes without any scaling approach needed. However, in our case the “elephant” moves and changes its behavior, e.g. with the diurnal cycle. Thus, ERF is a data-assimilation approach that attempts to recover the whole picture of “the moving elephant” using snapshots of varying parts of “the elephant” over space and time. We found that the fluxes of sensible heat, latent heat and CO2 integrated over the target region differed substantially from the tower observations in their expected value (+27%, −9%, and −17%) and spatio-temporal variation (−22%, −21%, and −3%, respectively) in 2011 July to Aug for WLEF television tower. ERF systematic uncertainties are bound within −11%, −1.5% and +16%, respectively, indicating that tower location bias might be even more pronounced than currently detectable.
For my undergoing work, Stefan, Ankur and I are working on applying ERF to the energy imbalance challenge lying in eddy covariance flux measurements. We are moving forward to reconsider mass conservation equation and expand ERF to storage flux and advective divergence terms in order to explain the energy imbalance closure (Metzger, 2016; Xu, Metzger, Desai 2015).
As you work more with flux data, what skills do you feel you need to acquire (either in the past or near future) to make your studies more effective?
Most times, I work with raw data. So I might have more experience in dealing with raw eddy covariance measurements. Before we can actually use the measured flux for scientific research, a list of corrections, preprocessing steps and quality tests are needed, e.g. planar fit, lag correction, despiking, stationarity test, etc. Usually, these steps have to be repeated many times due to numerous unexpected incidences, e.g. erroneous data and sensor malfunction, or adjusting selection criteria. Therefore, making processing codes modularized is very essential and efficient. Locating and adjusting a minor parameter through 500 lines of scripts and reprocessing it can take me half hour, while it only takes me less than 5 min change the input and re-run the function if the scripts are modularized.
If you are working with flux data that may not be processed by yourself or be processed by different teams, be cautious about the potentially different criteria people used to filter the data. Each tower team may set the criteria differently based on their research purposes. Blindly assuming all flux data are been processed and filtered similarly may lead to unexpected results .
What resources do you think the flux networks or communities have provided or could potentially provide to help your studies or career?
I think young scientist network get-together each year at the AGU is very helpful. Meeting and talking with peers, who are also working with flux data at the same early career stage, gives me some reference and coordinate on what I am working. This is way better than just sitting in front of computer myself debugging through flux codes and simply encouraging myself to move on. This is a very good opportunity to know other young scientists’ work and share our research experience.
Fluxnet is committed to publishing free and standardized flux data across hundreds of sites. Although currently I have only used several sites, the vast data source under different climates and ecosystems can be really invaluable for us to evaluate how well ERF works at different complex systems.
What are your career goals and aspirations?
Since I enjoy doing research as well as teaching students, I hope I can find a faculty position sometime somewhere to pursue these two interests. In the near future after my graduation, I want to find a postdoc position further studying surface-atmosphere exchange using eddy covariance measurements. I am particularly passionate about how heterogeneous surface exchange can affect the development of boundary layer; how flux towers can represent heterogeneous surface; the model-data comparison and assimilation among earth system models and ERF-projected flux maps; and ERF-projection as inversion prior for atmospheric inversions.
— Texts are provided by Ke Xu, Photos are provided by Johnathan Thom —
Reference:
- Metzger: Surface-atmosphere exchange in a box: Making it a suitable representation for in-situ observations, 32nd Conference on Agricultural and Forest Meteorology & 22nd Symposium on Boundary Layers and Turbulence & Third Conference on Biogeosciences, Salt Lake City, U.S.A., 20 – 24 June, 2016.
- Xu, K., Metzger, S., and Desai, A. R.: Mapping surface-atmosphere exchange by using environmental response function for both turbulent and storage eddy-covariance fluxes, 48th AGU annual Fall Meeting, San Francisco, U.S.A., 14 – 18 December, 2015.
- Xu, K., Metzger, S., Desai, A.R., 2017. Upscaling tower-observed turbulent exchange at fine spatio- temporal resolution using environmental response functions. Agricultural and Forest Meteorology, 232:10-22,doi:10.1016/j.agrformet.2016.07.019